What is "sophie ramp"?
Sophie ramp refers to a breakthrough in the field of machine learning that enables AI models to continuously learn and adapt to new data without the need for retraining. This transformative technology has the potential to revolutionize various industries by allowing AI systems to remain current and accurate even as the underlying data evolves.
Sophie ramp is named after the research team led by Sophie Le Meur at Google AI. The team developed a novel approach that leverages meta-learning techniques to train AI models that can effectively learn from new data without forgetting previously learned knowledge. This continuous learning capability sets Sophie ramp apart from traditional machine learning methods, which typically require extensive retraining when new data becomes available.
The importance of Sophie ramp lies in its ability to address the challenge of data drift, which occurs when the distribution of data changes over time. In real-world scenarios, data is constantly evolving, and traditional AI models can quickly become outdated as the underlying data shifts. Sophie ramp, however, enables AI models to adapt to these changes seamlessly, maintaining their accuracy and effectiveness even as the data landscape evolves.
The benefits of Sophie ramp extend to a wide range of applications, including:
Real-time decision-making: AI models can continuously learn from new data, allowing them to make more informed and accurate decisions in real-time scenarios. Fraud detection: AI systems can adapt to evolving fraud patterns, improving their ability to detect and prevent fraudulent activities. Predictive maintenance: AI models can continuously learn from sensor data, enabling them to predict and prevent equipment failures more effectively.While Sophie ramp is still a relatively new technology, it has the potential to transform the field of machine learning and drive innovation across various industries. By enabling AI models to continuously learn and adapt, Sophie ramp paves the way for more robust, reliable, and intelligent AI systems.
Sophie Ramp
Sophie ramp, a groundbreaking advancement in machine learning, empowers AI models with continuous learning capabilities, eliminating the need for retraining as data evolves. This transformative technology opens up a world of possibilities, offering key aspects that shape its significance:
- Continuous Adaptation: AI models seamlessly adapt to changing data distributions, maintaining accuracy over time.
- Real-Time Learning: Models can learn and make informed decisions based on the latest data, enabling real-time applications.
- Fraud Detection: AI systems can continuously learn evolving fraud patterns, enhancing their ability to detect and prevent fraudulent activities.
- Predictive Maintenance: Models can continuously learn from sensor data, predicting and preventing equipment failures more effectively.
- Data Drift Mitigation: Sophie ramp addresses the challenge of data drift, ensuring AI models remain relevant even as data changes.
- Meta-Learning Approach: The technology leverages meta-learning techniques to train AI models that can effectively learn from new data without forgetting previous knowledge.
- Broad Applicability: Sophie ramp has applications across various industries, including finance, healthcare, manufacturing, and retail.
In conclusion, Sophie ramp revolutionizes machine learning by enabling continuous learning and adaptation. Its key aspects empower AI models to handle evolving data, enhance decision-making, and drive innovation across industries. As technology advancements continue, Sophie ramp has the potential to shape the future of AI and its applications in the real world.
Continuous Adaptation
Sophie ramp's continuous adaptation capability empowers AI models to handle evolving data distributions, ensuring their accuracy over time. This is achieved through the use of meta-learning techniques, which enable models to learn how to learn from new data without forgetting previously learned knowledge.
- Facet 1: Handling Data Drift
Data drift occurs when the distribution of data changes over time, rendering traditional AI models outdated and inaccurate. Sophie ramp addresses this challenge by enabling models to continuously learn and adapt to these changes, maintaining their effectiveness even as the underlying data evolves.
- Facet 2: Real-Time Decision-Making
In real-time decision-making scenarios, AI models need to be able to adapt quickly to changing data conditions. Sophie ramp allows models to learn from new data in real time, enabling them to make more informed and accurate decisions even when the data landscape is constantly shifting.
- Facet 3: Fraud Detection
Fraudulent activities often exhibit evolving patterns. Sophie ramp's continuous adaptation capability enables AI systems to learn and adapt to these changing patterns, improving their ability to detect and prevent fraudulent transactions.
- Facet 4: Predictive Maintenance
Predictive maintenance systems rely on data to identify potential equipment failures and proactively prevent them. Sophie ramp allows these systems to continuously learn from sensor data, improving their ability to predict and prevent failures even as the operating conditions change.
In conclusion, Sophie ramp's continuous adaptation capability is a key aspect that sets it apart from traditional machine learning approaches. By enabling AI models to seamlessly adapt to changing data distributions, Sophie ramp paves the way for more robust, reliable, and accurate AI systems.
Real-Time Learning
Real-time learning is a critical component of Sophie ramp, enabling AI models to continuously learn from new data and make informed decisions based on the latest information available. This capability is essential for applications where timely and accurate decision-making is crucial.
One key example of real-time learning in Sophie ramp is in the context of fraud detection. Fraudulent activities often exhibit evolving patterns, and traditional AI models can quickly become outdated as these patterns change. Sophie ramp, however, allows AI systems to continuously learn and adapt to these changing patterns, improving their ability to detect and prevent fraudulent transactions in real time.
Another practical application of real-time learning in Sophie ramp is in the field of predictive maintenance. By continuously learning from sensor data, AI models can identify potential equipment failures and proactively prevent them, reducing downtime and maintenance costs. This capability is particularly valuable in industries such as manufacturing and transportation, where equipment failures can have significant consequences.
In summary, the real-time learning capability of Sophie ramp is a key factor in its ability to enable AI models to make informed decisions based on the latest data available. This capability is essential for applications where timely and accurate decision-making is critical, such as fraud detection and predictive maintenance.
Fraud Detection
Fraud detection is a critical aspect of financial and cybersecurity, and AI systems play a vital role in identifying and preventing fraudulent activities. Traditional AI models, however, can quickly become outdated as fraud patterns evolve over time. Sophie ramp addresses this challenge by enabling AI systems to continuously learn and adapt to evolving fraud patterns, significantly enhancing their ability to detect and prevent fraudulent activities.
Sophie ramp's continuous learning capability is particularly valuable in fraud detection because it allows AI models to identify and adapt to new fraud schemes that may not have been encountered during the initial training phase. This is crucial in combating sophisticated fraudsters who are constantly developing new and innovative ways to exploit vulnerabilities in systems.
One real-life example of Sophie ramp's effectiveness in fraud detection is in the financial sector. Banks and credit card companies use Sophie ramp-powered AI systems to monitor transactions and identify suspicious patterns that may indicate fraudulent activity. These systems continuously learn from new data, including historical fraud cases and emerging fraud trends, enabling them to detect and prevent fraud with greater accuracy and efficiency.
In summary, the connection between fraud detection and Sophie ramp is critical in the fight against fraud and financial crime. Sophie ramp's continuous learning capability empowers AI systems to adapt to evolving fraud patterns, enhancing their ability to detect and prevent fraudulent activities, and protecting individuals and organizations from financial losses.
Predictive Maintenance
Predictive maintenance, a key application of Sophie ramp, involves using AI models to analyze sensor data and predict potential equipment failures before they occur. This capability is crucial for industries such as manufacturing and transportation, where equipment downtime can lead to significant financial losses and safety hazards.
Sophie ramp's continuous learning capability plays a vital role in predictive maintenance by enabling AI models to continuously learn from new sensor data and adapt to changing operating conditions. This allows the models to identify subtle patterns and anomalies that may indicate an impending failure, even if these patterns were not present in the initial training data.
One real-life example of Sophie ramp's effectiveness in predictive maintenance is in the aviation industry. Airlines use Sophie ramp-powered AI systems to monitor aircraft engines and other critical components. These systems continuously learn from sensor data collected during flights, enabling them to predict potential failures with greater accuracy and provide timely alerts for maintenance.
In summary, the connection between predictive maintenance and Sophie ramp is critical for ensuring the reliability and safety of equipment in various industries. Sophie ramp's continuous learning capability empowers AI models to continuously adapt to changing operating conditions and identify potential failures before they occur, reducing downtime, maintenance costs, and safety risks.
Data Drift Mitigation
Data drift is a common challenge in machine learning, where the distribution of data changes over time, rendering AI models outdated and inaccurate. Sophie ramp tackles this challenge by enabling AI models to continuously learn and adapt to evolving data distributions, ensuring their relevance and effectiveness even as the underlying data changes.
One real-life example of Sophie ramp's effectiveness in data drift mitigation is in the healthcare industry. AI models used for disease diagnosis and treatment planning need to adapt to changing patient demographics, medical advancements, and new disease strains. Sophie ramp allows these models to continuously learn from new patient data, ensuring they remain accurate and reliable over time.
The connection between data drift mitigation and Sophie ramp is crucial for the long-term effectiveness of AI systems. By addressing the challenge of data drift, Sophie ramp ensures that AI models can continuously adapt to changing data landscapes, making them more robust and reliable in real-world applications.
Meta-Learning Approach
The meta-learning approach is a fundamental component of Sophie ramp, enabling AI models to continuously learn and adapt to evolving data distributions. Meta-learning techniques empower models to learn how to learn, allowing them to effectively learn from new data without forgetting previously acquired knowledge.
One real-life example of the practical significance of the meta-learning approach in Sophie ramp is in the field of natural language processing (NLP). NLP models trained using Sophie ramp's meta-learning techniques have demonstrated the ability to learn new languages and tasks more quickly and efficiently than traditional AI models. This capability is crucial for developing AI systems that can handle a wide range of language-based applications, such as machine translation, text summarization, and question answering.
In summary, the meta-learning approach is a key aspect of Sophie ramp that enables AI models to continuously learn and adapt to changing data distributions. This capability is essential for building robust and reliable AI systems that can handle real-world applications where data is constantly evolving.
Broad Applicability
Sophie ramp's broad applicability stems from its ability to empower AI models with continuous learning capabilities, making it a valuable tool across a diverse range of industries.
- Finance:
In the financial sector, Sophie ramp enhances fraud detection systems by enabling AI models to continuously learn and adapt to evolving fraud patterns. This helps financial institutions identify and prevent fraudulent transactions more effectively, protecting their customers and assets.
- Healthcare:
Within the healthcare industry, Sophie ramp improves the accuracy of disease diagnosis and treatment planning. AI models trained with Sophie ramp can continuously learn from new patient data, adapting to changing patient demographics, medical advancements, and new disease strains.
- Manufacturing:
In manufacturing, Sophie ramp enhances predictive maintenance systems, enabling AI models to continuously learn from sensor data and identify potential equipment failures before they occur. This helps prevent unplanned downtime, reduces maintenance costs, and improves overall equipment effectiveness.
- Retail:
Within the retail sector, Sophie ramp improves demand forecasting and inventory management systems. AI models trained with Sophie ramp can continuously learn from sales data and customer behavior, enabling retailers to optimize their inventory levels and meet customer demand more effectively.
In conclusion, Sophie ramp's broad applicability across industries is driven by its ability to enhance the performance and reliability of AI models in real-world applications where data is constantly evolving. By continuously learning and adapting, Sophie ramp enables AI models to handle a wide range of tasks and challenges, leading to improved outcomes and increased efficiency in various domains.
Frequently Asked Questions about Sophie Ramp
This section addresses some commonly asked questions about Sophie ramp, providing brief and informative answers to clarify any misconceptions or concerns.
Question 1: What is the significance of Sophie ramp's continuous learning capability?
Answer: Sophie ramp's continuous learning capability empowers AI models to adapt to evolving data distributions, ensuring their accuracy and effectiveness even as the underlying data changes. This addresses the challenge of data drift and enables AI models to handle real-world applications where data is constantly changing.
Question 2: How does Sophie ramp benefit industries beyond fraud detection and predictive maintenance?
Answer: Sophie ramp has broad applicability across various industries, including finance, healthcare, manufacturing, and retail. Its continuous learning capability enhances AI models' performance in tasks such as demand forecasting, inventory management, disease diagnosis, and treatment planning, leading to improved outcomes and increased efficiency.
In summary, Sophie ramp's continuous learning capability is a key aspect that sets it apart from traditional machine learning approaches. This capability enables AI models to adapt to changing data distributions, making them more robust, reliable, and applicable across a wide range of industries.
Conclusion
Sophie ramp represents a significant breakthrough in the field of machine learning, empowering AI models with continuous learning capabilities. By addressing the challenge of data drift, Sophie ramp ensures the accuracy and effectiveness of AI models even as the underlying data evolves. This continuous learning capability opens up new possibilities for AI applications across a wide range of industries.
While Sophie ramp is still a relatively new technology, its potential impact is immense. As AI becomes increasingly integrated into our lives, the need for robust and adaptable AI systems will only grow. Sophie ramp provides a path forward for the development of such systems, enabling AI to handle the complexities and uncertainties of the real world.

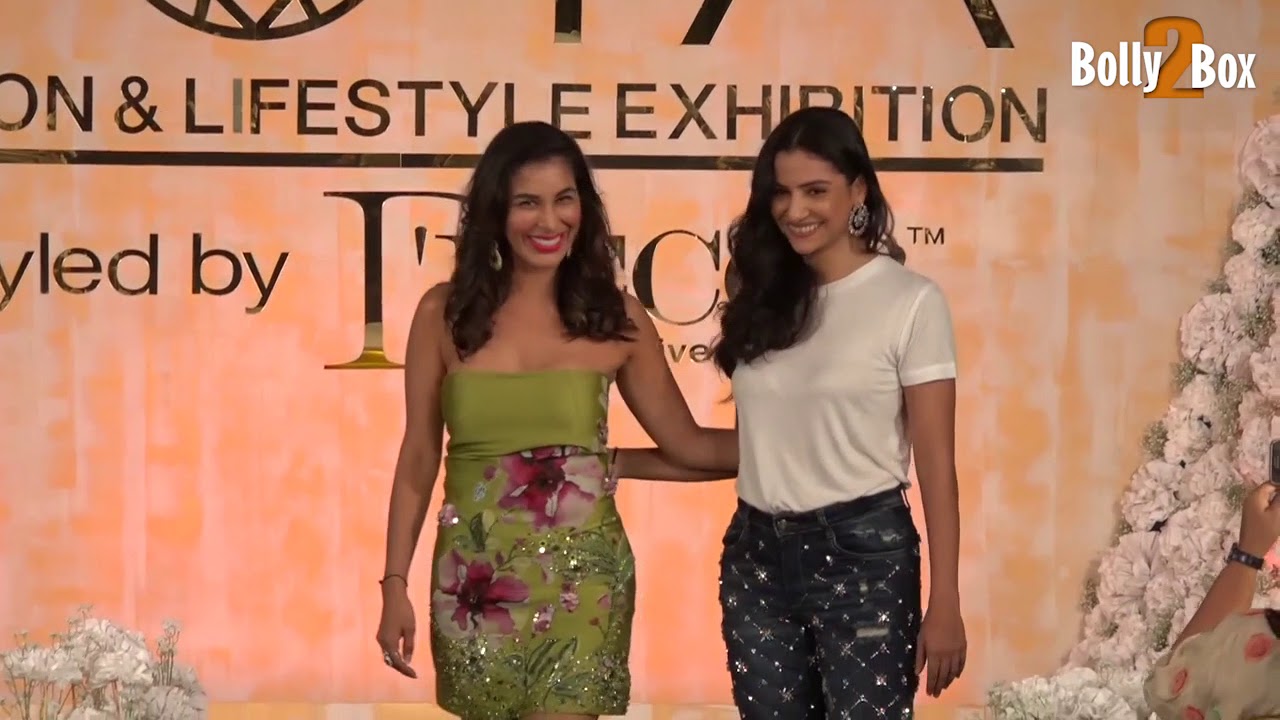